Better eQTLs for integrative functional genomics? – discussion on SMR/HEIDI
This is a follow-up post to the mini-review of the work of Zhu et al. [1] and is a reaction to tweet from @JosephPowell_UQ 🙂
In short, in Zhu et al. [1] have used eQTL results from peripheral blood to answer the question of potential biological function affected by genetic variation associated with five complex trait. Using such eQTLs may be not ideal, although very understandable (see comment [5] of mini-review); to this @JosephPowell_UQ commented
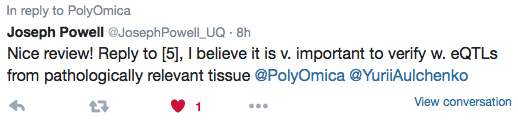
It is worth to note that in case one would be willing to do SMR/HEIDI analyses in relevant tissues (and/or under relevant conditions, e.g. ‘response eQTLs’) (s)he would face the problem that not much is available yet in terms of sample sizes. There are systematic resources such as GTEx, and much is published elsewhere on specific tissues, however, unless this is blood, sample sizes are typically in order of (few) hundreds.
How much of concern is that? – typically, eQTLs are rather strong so that even on 100s of samples we see 1000s cis-e QTLs. Looking at the formula for SMR, it is expressed as function of Z-test value, in that having descent Z should give descent power. May be current samples are enough to at least give it a try? (we at PolyOmica will definitely do that) …
On a sobering note, the authors of [1] did look up schizophrenia loci on brain eQTLs [2], but found relatively little. Why? First, it looks like in the brain eQTL data they use (only 134 individuals) they did not see many eQTLs. Next, the variation of expression between brain regions is large, so one may say that averaging expression across 10 brain regions [2] does not really give the ‘proper tissue’ (and one could also claim it was not proper ‘stage of development’, yes, one always can…). So yes, it feels that this analysis was limited by availability and size of tissues-specific eQTL resources.
This brings us to an interesting question – given limited resources, should one go for more specificity (e.g. all kind of different tissues, stages of development, conditions) or go for bigger sample size at limited number of tissues/stages/conditions? The argument for doing former is plain biology while the argument for doing latter is consideration that with really big sample sizes we should be able to see some association even when we are not measuring at exactly the time point at exactly the condition – just because in really big sample some people will be at ‘right’ conditions; that even if the exact right tissue is not measured, other tissues may provide a proxy. This reasoning is similar to that we successfully apply for complex trait GWAS. At the end, this is about costs and benefits and both need to be done. Having both specific and large resources (ideally, but not necessarily as a single resource) would probably allow us figuring out proxies and signatures which easily available tissues carry about more specific tissues and events.
So… We will love to see bigger and more specific eQTL resources!
[1] Zhu et al., Integration of summary data from GWAS and eQTL studies predicts complex trait gene targets. doi:10.1038/ng.3538
[2] Ramasamy eQTL data, 134 individuals, gene expression averaged across 10 brain regions (see online methods in [1]). Worth to note, in brain, the authors of [1] only followed up loci which passed SMR/HEIDI on blood. We guess this indicates that at least for loci studied the brain eQTLs were not super-strong ‘per se’ unless the blood information was used to prioritise them.
1 Comment
Jian Yang
10 August 2016 at 05:08 •Hi Yurii,
Thanks very much for the nice summary of our paper. I have added two links at the CTG forum to these two posts.
http://gcta.freeforums.net/thread/305/nice-summary-smr-yurii-aulchenko
Regarding the Ramasamy eQTL data, we didn’t perform a genome scan using this data set because we were only able to access the data for a few specified genomic regions. Of course, as you have commented, power is also an issue given the small sample size.
Cheers,
Jian Yang